Transcript
Is your small business growing faster than you can keep up with? It might be time to grow your team, document key processes, and make sure you’re using the right technologies to scale up operations. In other words, your company might need business infrastructure. At Equilibria, Incorporated we specialize in building business infrastructure.
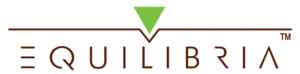
That’s why we’re proud to sponsor Season 22 of the Business Infrastructure podcast which features an AI audio conference. Our goal is for you to learn some important tips, strategies, and tactics to help you cure any back-office blues you might be experiencing while also advancing your knowledge of business infrastructure.
This is Episode 279 – Exploring the Frontiers of AI for Small Businesses with Dean Hamilton.
Welcome back everyone! It’s good to see you all again. Hard to believe, but we’re already at the midway point of our AI audio conference.
So far, you’ve heard our opening keynote speaker as well as five other speakers, each of whom demonstrated the AI tools they use to streamline their business operations. Now you’re going to hear from another keynote speaker.
This speaker is no stranger to this podcast and he’s definitely not a stranger to technology. In fact, he has over 30 years of experience as a technologist. It’s none other than…Dean Hamilton!
Dean is a Partner and CTO at Wilson Perumal & Company – a leading management and strategy consulting firm. He was once included in a list by Forbes.com of “The Top 8 Technology CEOs to Watch.” His areas of expertise include technology, business strategy and product innovation, design, development, and delivery. You’re about to hear his predictions on what the future holds for small businesses, AI, and its ability to transform the way your business operates.
Dean will be interviewed during this keynote by the host and producer of the Business Infrastructure podcast, Ms. Alicia Butler Pierre.
Please welcome to the stage, Dean Hamilton and Alicia Butler Pierre!
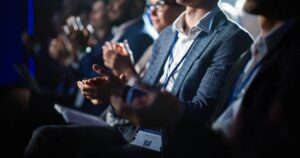
Thank you again for such a lovely introduction, Kenya! Dean Hamilton…it’s always a pleasure seeing you and speaking with you. How are you?
I’m well. Thanks for having me again, Alicia.
Whenever I think of making processes flow more efficiently through automation, you’re the first person that comes to mind! The last time you came onto the show, you talked specifically about robotic process automation or RPA and how there’s a continuum if you will. Can we start there? Why do you describe it as a continuum and what role does AI specifically play as it relates to that continuum?
It’s a good question. I think RPA can go from sort of very simple automation of repetitive processes, which is, I would say, sort of the sweet spot of traditional RPA. So, you’ve got something that you’re just doing over and over again. Maybe it’s what we call a swivel chair application. You’re opening one application, you’re clicking on a few things, you’re cutting and pasting, you’re opening another application. But it’s a very deterministic, repetitive workflow. And so traditional RPA has lent itself very well to doing that.
But traditional RPA didn’t, at least historically, lend itself very well to anything that needed a lot of subjective human reasoning, right? You couldn’t reduce it to an algorithm. Somebody had to really think about it and maybe make lots of exception cases. So, there’s lots of subjectivity in the decision-making. I think where AI starts to kind of come and impinge on that is, if you, for instance, have a lot of data, if you’ve had humans doing that process, and the process has been instrumented, you’ve been able to collect a fair amount of data about how humans make those decisions, then you can have a machine learning algorithm learn from that data and be able to kind of infer the reasoning that the humans would make.
So, for the part of the process that’s very repetitive you just kind of handle that the old-fashioned way. And for the part of the process that is the exception cases and perhaps needs human reasoning instead of like the old AI, where you would basically have to farm it out to a real human. Well, you’d farm it out to an AI who would have basically the reasoning of a lot of humans who have done that work before, captured in its model.
And you would give it whatever you need to ask it and it would give you your response back. Like if you had a human sort of sitting in a kind of a supervisory mode, if you will, over the AI to handle the exception cases. So, I think sometimes that’s referred to as sort of cognitive AI and there are all kinds of weird terms that are being bandied about for how things like AI and traditional automation work together.
So don’t get too hung up on that term. But I think sort of the future is this collaboration between traditional sort of programmatic automation that is kind of doing the highly repetitive things and then sort of the data science-driven forms of automation. Which is
kind of going to be based on your sort of a machine learning model and an inference engine that can then really do the highly subjective reasoning to do difficult things. Does that make sense?
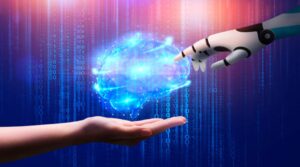
It does. But to be sure, I’d like to describe a typical scenario for many small business owners to make sure we understand what you just said. Earlier in the conference, we had a speaker, Aurelien Coq, who described the Order-to-Cash process and how Esker AI can help automate that process. If we wrote the steps of our process to accept orders and payment for those orders, there might be several steps that are repetitive. Those steps could potentially be a candidate for RPA, right?
Sort of traditional RPA.
Okay, traditional RPA. But let’s say there’s a point in that same Order-to-Cash process where something goes wrong. For example, when a customer pays with a credit card, that credit card is declined or if a customer is late making a payment. Both of those scenarios require a level of, as you described it, subjective or human reasoning as to how to best handle it. Are these exceptions where that “cognitive” AI that you described could be used?
I think it depends on what kinds of exceptions, right? I mean, there are some exceptions that are yes, they are exceptions, but you don’t have a lot of exceptions cases to deal with. And the ones that you have to, it’s pretty clear what you do with that exception. So, I think you can take a kind of automation approach to even handling those exceptions. You can program those exception cases using traditional programmatic forms of automation.
But I think when the exceptions really require human reasoning, it’s not very clear what to do. Okay, that’s usually when we kind of say, Well, some human being has to make a judgment call on this, right? Well, then if you have lots of data that can show how humans in the past have perhaps made judgment calls about similar things, then you can teach or train a model to kind of infer how we think about those decisions and do it for you. It won’t be 100% right. It may be 90% right, but it’ll capture a significant part of our subjective capability to reason about that issue.
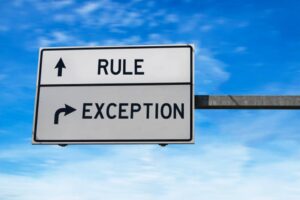
Okay, I think I got it! So, if a customer is late paying it could trigger a collections process which, to your point, would still be a candidate for traditional RPA. But, if that customer is late paying because, let’s say, that customer went out of business then that is a true exception that requires more thinking, more cognitive thought. That’s a candidate for AI. But in order for the AI to work, we have to feed it several data points that involve troubleshooting true exceptions to the process so that it can make inferences. What are some AI tools, Dean, that us small business owners can use to build such a database? Can we use something like Chat GPT to upload our own exceptions handling data?
Yeah, I mean, the two approaches. One is the machine learning approach that assumes that you have your own internal data about how those decisions have been made. And so basically, you’re just kind of capturing in the machine learning model, the AI, how
your own people have handled that thinking and that reasoning.
If you don’t have that, then what you’re alluding to more is that, well, can you perhaps from a larger universe of reasoning that is captured in, say, a public foundation model, something like a Chat GPT, can you get that model to sort of think about the problem and make a recommendation to you? And I think that’s becoming a very real use case as these foundation models become more and more powerful and capture more and more sort of general-purpose knowledge, about how we, as human beings, think about things.
Now, one of the things I particularly enjoy about our conversations is your ability to break down very complex topics into information that is easy to understand and relatable. With that in mind Dean, how can we as small business owners evaluate whether AI can really help us in some of our day-to-day back-office operations?
Yeah, I think that’s a great question, and I don’t know that I have a perfect answer to it because I think, well, first of all, AI is just this broad universe of things, right? Going all the way from old school expert systems to kind of regression and classification algorithms all the way to this sort of newer kind of neural networking based, generative AI stuff that’s all the rage now there’s like a vast, vast range of capability that’s covered by that term “AI” and sort of the legacy kind of AI where you’re essentially creating a function, a model that fits some data that you have usually required you to go out and actually have a lot of data and train the model, right?
So, you have to show the model a lot of things and then it would kind of figure out how to replicate, reproduce those patterns so that then you can show it something new and it will give you a reasonable inference. But I think what’s different now that is with these,
especially these large language foundation models, is these things are so capable of they’ve captured so much human reasoning on so many subjects because of just how much information they were trained on that I think it’s now possible for small businesspeople to actually go to those models and get them to help with certain problems.
And instead of having to fully train a Chat GPT, which would be beyond the resources of any small or even medium-sized business, you can now sort of take these foundation models and do what’s called one-shot training or multi-shot training. And essentially what it is basically by you just kind of having a conversation, in a way, with the model and perhaps feeding the model some examples about something specific about your business.
There’s something specific about Alicia’s business, and you want the model to understand that secret sauce that you do well, right? You only do well. You don’t have to start over. You can build on the model’s large corpus of data that it’s been trained on, that’s given it its reasoning capacity, and then you can start to sort of tell it the incremental difference. What else is it that you want to teach it about your small business? And now suddenly you’ve got what’s called a fine-tuned model, and that finetuned model now knows about your business.
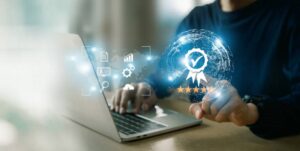
It wasn’t really very costly to fine-tune it because you didn’t have to go train it from scratch. And now you can start to use that fine-tuned model in some way. So, I do think these foundation models now really kind of democratize a lot of capabilities that were otherwise in AI capabilities would have just been completely inaccessible to small businesses. Now there’s a whole bunch of risks also that we have to recognize that these things are not perfect, and they make a lot of mistakes, and they make up stuff, and they call them hallucinations. I sort of hate that word because it almost sort of anthropomorphizes these models. And these models are not people.
They are actually algorithms, but they make significant mistakes. And I think the biggest challenge that all business face right now, whether it’s a small business or big business with where AI is going, is how do you build trust in what the AI is telling you? How can I
know? Because if I’m going to base my business decisions on it, I have to trust it. Now, I can trust you, Alicia. I know you’re not going to be perfect. You are going to make mistakes, but I have built a relationship with you to kind of know that in this certain area, I can trust. If I give Alicia this problem and she tells me this is what I should go left instead of right.
She’s thinking about that the right way. I know she understands what I told her. I think that’s still the big challenge because these models sort of lack transparency, that is, to tell us how they were trained, what kinds of biases, what kinds of gaps in training they have. And they lack explainability. That is if, Alicia, you say, “Go left instead of right,” and I say to you, “Why?” Well, you’ll give me a reasoned explanation for that and then I can decide whether that makes sense to me.
The models are still developing the capability to do that, to basically say, “Hey, I’m not just telling you this is what you should do, but I’m going to be able to explain to you why this is what is the right thing to do, and I’m going to be able to sort of bring the receipts too. I’m going to be able to show you what my reasoning is based on.” And I that’s coming along, the pace of development of this stuff is really quite stunning. But I think until those capabilities are mature, especially for small businesspeople who are relatively unsophisticated, they’re going to have a hard time knowing how to trust these models. I think that’s going to be the biggest problem.
So I guess it’s safe to say that many of us entrepreneurs may not be early adopters of using some of these AI tools to streamline our back-office operations.
Unfortunately, I think the truth is many of you will be early adopters and will feel a lot of pain from being early adopters.
Well, the truth is Dean, it’s hard to not take notice of AI because it’s everywhere right now. It’s ubiquitous. It’s interesting listening to you talk about how these AI models currently lack transparency and explainability right now, but we know it will get better. But as the technology gets better, so will the bad actors. If you had a crystal ball, what would be your prediction for the immediate future of AI and the way we operate our small businesses?
Yeah. I hate to say this, but I think the sort of destructive use cases, at least in the short term, will far outweigh the constructive use cases. In other words, I feel the bad actors and their ability to rapidly exploit AI in ways that increase risk for businesses. Just think
about it. Right now, on the dark web, there’s a service called Fraud GPT that will write socially engineered hacking attacks for you using the latest social engineering methods that can evade the detection of all of the protections that we have in place.
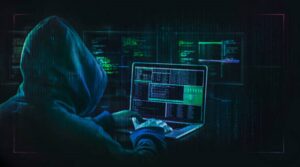
That’s where the frontier of use, unfortunately, of this stuff is going to be. And that’s where we are least prepared, I think, as businesses or even as individuals, to really kind of protect ourselves against these deep fakes and all sorts of kind of novel ways of exploiting us, exploiting all of the public information that is online about us, whether it’s in the social networking areas and so on, there’s just going to be a massive, massive set of innovation.
Unfortunately, that is going to be very difficult, I think, for organizations to kind of cope with. So, I sort of feel like the first place organizations need to focus when it comes to AI is how we can start to get an organized and disciplined set of practices within our
organization about how we’re going to try to protect ourselves from the malicious use cases. What are the malicious use cases? How could they affect us there? Of course, they’re evolving.
We can’t predict all of them because I think the risk scenarios are much larger than the benefit scenarios. And I know everybody writes about the benefit scenarios and I know all the great articles you read about all the wonderful things that it can do for your
business, and I think it has the promise to do many of those things. But before those things are real, there’s a promise that it’s going to do a lot of destructive things that you’re going to be ill-prepared to manage.
And I appreciate you talking about that because you’re right, it’s so easy to become enamored with AI and get caught up in that shiny object syndrome without having an understanding of the risks that are involved.
Yeah. And you also have to remember that with any technology, especially new technologies, it’s very easy to do pilots. Pilots are kind of these 80/20 rules, right? It’ll take you 20% of the time to get to 80% of the capability with a pilot and then it’ll be really exciting. Look at how quickly we were able to get that AI to do this thing for us. But that other 20% of the way to really make it actually fit for your use case can take you to 80% of the time.
That’s the difficult part, right, going from pilot to production. And to go to production, you’ve got to handle all of those security cases and risk cases and all of that. The pilot, you didn’t think about that. You were just showing the Wow, yeah, this capability is kind of there to do this. Wow, that’s really interesting. Now how do I make it so that my business can use it and rely on it day in, day out and not suffer any negative consequences? That’s hard.
That is hard, especially because as you mentioned, small businesses don’t have the financial resources as large enterprises. I went to a cybersecurity conference not too long ago and some vendors did a search about me on the dark web. A lot of the information was either old or inaccurate but some of it was accurate. I remember being, 1) angry that my personal information was out there potentially in the wrong hands and 2) scared.
But I know you’re not sharing this information with the intent to instill fear in us. You want to educate and encourage us. What I’m hearing you say is, “Don’t just dive in. Do some research first and really figure out if the AI tool you’re thinking of using will be more harmful or helpful to the way you operate your business.”
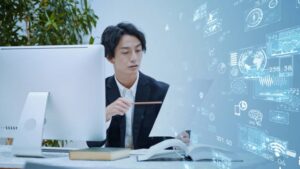
Yeah, that’s right. And develop a plan. Don’t just look at the bright, shiny use cases, but have a realistic view. I think everybody should think about some sort of risk framework that they can operate within. What are the risk tolerances of my business or a particular
use case that I’m thinking about using this stuff for? Some applications the risks are going to be very low.
The risk is just asking yourself what’s the worst thing that could happen? And there are going to be some use cases where the worst thing that can happen isn’t all that bad, and the benefits outweigh those risks. And so, it’s worth dabbling and moving forward and experimenting and learning because your competitors are going to do that. And you’re going to have to be prepared to sort of keep pace with what’s happening.
But it’s also really important to understand the other side of that, that there are going to be some risks that if you’re not thinking about them seriously and you’re not anticipating them, they can actually destroy your business or destroy your business’s reputation.
You went chasing after this nice little EBITDA benefit that you saw out there, but you created this huge existential risk for your business, and nobody wants to do that. So, you have to be able to sort of think constructively and intentionally about those risk issues to really come up with a good plan for how to really get the benefits and avoid the risks. Does that make sense?
Yes, it does Dean. This has been a phenomenal conversation and I love the consultative approach you used in all of your responses. You were careful to remain agnostic about any particular AI tool and instead focus on the technology itself – the good, the bad, and the ugly. I can’t thank you enough! Ladies and gentlemen, please join me in thanking Dean Hamilton for this enlightening conversation!
Thanks for having me!
Thank you for listening! If you enjoyed this episode, then please subscribe and give us a five-star rating and review. You can watch the video version of this keynote interview, as well as access links to the resources Dean mentioned, at BusinessInfrastructure.TV. That’s BusinessInfrastructure.TV.
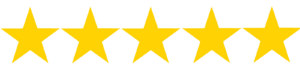
Coming up in the next episode we will resume our AI product demo sessions.
Be sure to come back to the place wherever you’re listening to this episode, so that you don’t miss that demo! Until then, remember to stay focused and be encouraged. This entrepreneurial journey is a marathon and not a sprint.
Alicia Butler Pierre hosted and produced this episode. Opening and closing voiceovers by me, Kenya A. Moses. Audio editing by Olanrewaju Adeyemo. Original score and sound design by Sabor! Music Enterprises. Video editing by Gladiola Films. A special thank you to Grant Revilla for creating the show notes.
This is the Business Infrastructure – Curing Back-Office Blues podcast.