Transcript
I’m Alicia Butler Pierre and this is Season 22 of the Business Infrastructure podcast. On this show we share operational tips, strategies, and tactics to help you cure any back-office blues you might be experiencing.
If you’ve been listening to this show for a while, then you know we’re always trying something different. And this season is no different. Through our partnership with Equilibria, Inc., we’re proud to bring you an audio version of our AI conference.
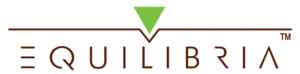
Over the next 12 episodes you’ll hear a combination of fireside chats, keynote speeches, and product demonstrations all centered around AI. I’ll be right there with you as you listen to each episode, hopefully asking the different speakers the questions you would want the answers to. Through this information, you’ll discover ways that you can leverage this technology to improve the way you operate your business. So, without
further ado, let’s start this virtual AI conference!
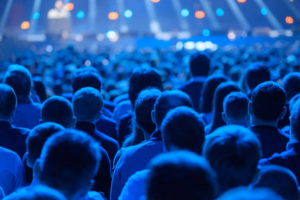
This is Ep. 273: Demystifying AI – a Fireside Chat with Kjell Carlsson
Welcome to the Business Infrastructure Podcast’s AI Conference! How’s everybody doing today? I’m Kenya Moses and it’s my honor to kick off this conference by introducing our first speaker.
Kjell Carlsson is the Head of Data Science Strategy & Evangelism at Domino Data Lab. He advises enterprises on how to drive business outcomes with AI and data science. His unique background in economics, strategy, management consulting, data science, and AI makes him well-qualified to give us a primer on AI. Specifically, what is it, why does it matter, and how can we use it to improve the way we operate our businesses?
Kjell will be interviewed in this fireside chat by the host and producer of the Business Infrastructure podcast, Ms. Alicia Butler Pierre.
Please join me in welcoming Kjell Carlsson and Alicia Butler Pierre!
Thank you for that lovely introduction and thank you everyone for joining us! Kjell, it’s a pleasure to be here with you to have this conversation to kick off our virtual AI conference. Let’s get right into it. Now, you describe yourself as an AI strategist and evangelist. What exactly does that mean?
Well, I have the incredible good fortune in the roles that I’ve been doing for a couple of years now to be in that role of advising folks on how they can leverage these nebulous, often untested, often unclear technologies to drive business impact. So, in my current role, head of Data Science Strategy at Domino, I do this with large companies in the pharmaceuticals, insurance, and healthcare realm.
A lot of folks who have sometimes hundreds, if not thousands of data scientists and are doing incredible things. But prior to that, I was an industry analyst at Forrester where I got to cover the full breadth of the AI data science, machine learning, computer vision space. So, it’s one where I get to be part advisor and consultant to help folks think about scaling teams and providing them with the technologies that they need access to.
So, it sounds like what you just said covers your role as an AI strategist, but what about your role as an AI evangelist?
There are so many incredible things that we can and should be doing with these technologies and that we should have been doing for a really long time now for a lot of them. And so, I get to stand on a soapbox there, but I also get to be a therapist. There are just so many folks who struggle with these technologies. They’re data science teams where the organization doesn’t understand them. They are being asked to do the impossible. They don’t have the resources that they need and it’s often helping guide them through it, realize that they’re not alone in this challenge, and help them see the light at the end of the tunnel and get there a little bit faster.
Ah, okay that makes sense. Now, Kjell, something else that I find really interesting about your background is that you have a doctorate degree from Harvard in economics. How did you go from studying economics to eventually becoming a data scientist?
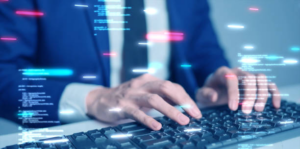
Well, an interesting story in all of this was how I became an economist, which was I was two courses away from a degree in computer science and I came to a course titled Artificial Intelligence. This was in the late ‘90s. I looked at the syllabus and syllabus said neural networks and heuristics and I thought, Nobody’s going to be using those anytime soon. They’ve been around for ages, and they haven’t gotten anywhere versus those economists over there are leveraging applied statistics and machine learning in order to pull really interesting insights from data. I can go in and do practical stuff with machine learning as an economist in a way that I’m not getting to do as a computer scientist.
So, the irony is entirely on me that I’m this person who went and dropped these courses way back when. And pretty much everything that I’ve done since about 2014, 2015 onwards has solely been within the AI space. But the big change happened around 2013, 2014 when I was put in charge of a data science team, and I had incredible data scientists underneath me who
came up with these incredible ideas. Amongst other ones were leveraging machine learning technologies to go in and understand what people were saying and to try and help them learn faster. So we were building AI applications that our BDRs and SDRs would be using to try and learn how to sell the product. We would ask them, “Okay, you just watched a training video on the product, you know, tell me about the product in your own words.”
And we would go in and transcribe that audio, listen to it, identify what it was that they were talking about, and saying, “Well, you missed these key value propositions, why don’t you try it again?” And so we started building those around that time. I eventually got poached and became an industry analyst covering the AI space and obviously it has just taken off since then. Again, I keep on pinching myself that I have the good fortune to have this role, but it does also drive home that you don’t have to go back that many years and the role of a data scientist didn’t exist.
That’s an interesting point, and I’m curious, Kjell, are data science and AI similar? I also heard you talk about machine learning. Is that a sort of branch of AI or is it the other way around, or…is it along a spectrum of some sort?
Well, that is one of the key things when it comes to Artificial Intelligence, is getting our terminology right.
Yes, please!
You’ll walk into a room and people will have all of these different opinions around Artificial Intelligence, and they usually be right. They were just talking about different elements, different parts of the spectrum. So, the folks who are complete detractors are absolutely right, but around certain particular aspects of it. And the folks who are gung-ho enthusiasts are usually absolutely
right, but around particular elements of it. And they never really overcome that lack of common vocabulary. But, yeah, Artificial Intelligence, back in the days, it used to refer to a whole host of different technologies.
Applied statistics was in there, and business rules engines were in there. But these days, when you say Artificial Intelligence, really folks are referring to a very specific branch of machine learning, and those are called artificial neural networks. And so, these artificial neurons are mathematical representations of the biological neurons in our brains. They take a couple of inputs, they
process them, they pass it on. And when you create millions, sometimes billions of these artificial neurons, usually millions, they can do incredible things in terms of detecting patterns in data and being able to replicate a lot of very human-like behaviors. So, these days, when folks are saying Artificial Intelligence, they’re really meaning artificial neural networks, which is the same thing as another term called deep learning.
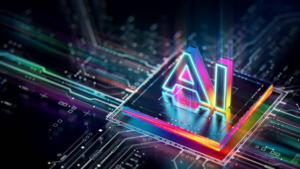
Deep learning is just these artificial neural networks, but they’re stacked in lots and lots of layers, hence it’s deep. But what’s happening right now is something called generative AI. You might have heard the term “large language models,” you might have heard the term “foundation models,” all referring to the same thing. It’s a particular type of artificial neural network, or particular type of deep learning, if you so will, which are, again, all different types of machine learning. And these are incredibly powerful. These are the things that if you’ve been playing around with Chat GPT, or you’ve heard of Chat GPT, these are the kinds of models that are making that possible.
The audience doesn’t know this Kjell, but when we were backstage talking, we were kind of chuckling over the fact that these technologies you’ve just described…they’ve been around for a while. So, it begs the question – why now? Why all of the hype? And please, be honest with us here, okay – is it that there were a bunch of data scientists who congregated under the cover of night, tinkering in a lab coming up with this stuff and then it…poof! somehow it got released into the universe?
Well, it is, to a certain extent, what you’re describing. There was a very specific invention in 2017. Deep learning has been around for a long time. Artificial neural networks have been around forever. I mean, they’re first proposed in the 1940s. But what happened in 2017 was the creation of something called the Transformer Network. It’s sort of based off of humans. When you think
of a word, the meaning of that word depends not just on the words that I’ve just said prior to the word.
It actually will change depending on what I then say next. So, the meaning of what I’m saying varies depending on looking just slightly forward and slightly backward in terms of a series, in terms of data, in terms of language, or something like that. So, in 2017, folks came up with this idea and said, “Hey, wait a minute, why don’t we try this and see whether or not our models work better?”
And it really transformed everything. It made it possible now to be able to analyze unstructured data like language, voice, images, and video in a way that we’ve never really been able to do before. So, there was this sort of spark, this AHA moment. Think back to 2001: A Space Odyssey, when the apes are learning how to use tools. We had those tools, but this one development really made it possible now for these models to behave in much, much more humanlike behaviors in a way that well, we had made some headway already.
Computer vision was already going like gangbusters. Facial recognition was taking off, but we hadn’t really cracked the nut around language and images. And so that happened in 2017. But then you can ask, Well, but that was five, six years ago. What on earth happened? Why did it take so long? Well, in the back rooms amongst data scientists, folks were playing with these and thinking, these things do incredible things.
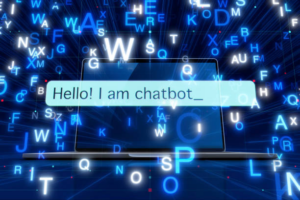
But they were the only ones who heard about it. They mentioned it upwards, and people were like, Yeah, whatever. That sounds like a science project. Let’s go and focus on creating those lead score models and other things that are actually going to drive us business soon. What happened then was November 2022, when Chat GPT came along and created a killer application around
this. They created an experience that anybody could go in and use and say, “Oh my goodness, this thing can do incredible things! I want to go in and write a resume for myself.” Voila! it will create a resume for me. “I want to create a better version of my existing resume.” Voila! it will do that. “I want to go in and create a performance evaluation for myself to give to my manager.” Voila! it will come up with one of those.
“I want to go in and write code without necessarily knowing what the syntax is” and things like that. Voila! it’s giving me code that I can then go in and execute or run an analytics query with. So that brought home to everybody, whether it be your children, grandparents, certainly everybody within the business that, Oh my goodness, this is real and this is very humanlike in terms of what it can do. And so that has then been the AHA moment. There’s a little bit of a repeat of 2012 when Siri and Alexa came around and for a little bit exec were like, Oh look, but AI must be real now because my Alexa device can speak to me.
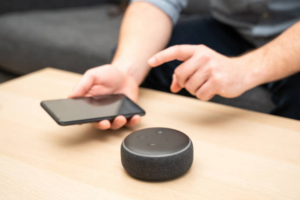
But that faded very quickly because really, we were very limited in terms of what capabilities it had there. Now really, it’s almost like you’ve cracked open Pandora’s box or the sky’s the limit, depending on how you view it, in terms of the kinds of use cases we can apply this technology to.
Speaking of use cases, I know when I first started using Chat GPT I was blown away, both by the speed of the response to my queries and the accuracy, although it didn’t always generate accurate information. And that’s the keyword I’d like for you to talk more about – “generate.” Specifically, “generative AI.” What exactly does that mean, Kjell?
So, as we were talking about before, there were these artificial neural networks. And for the most part, they’re really good at understanding unstructured data. But it’s mostly on the understanding of it. So, you can feed it a set of emails and it will understand: Okay, well, out of these emails, which of these are customers who are really unhappy with the product? What are they unhappy about? Or it’ll be like, Which of these ones have an intent to purchase my product? Or which ones of these are going to go and give me a very bad net promoter score? So, most of the way that we’ve used these technologies in the past has been in the analysis or in the prediction, What is this person going to do? What are the characteristics of this person?
Now, what generative AI is, is you can take that same model and flip it on its head and say, Now instead of just going and telling me whether in this email this person was unhappy, I want you to create that email but one in which this person is now myself, and I am looking to go in and have a meeting with somebody. I’m looking to try and persuade somebody that they should take a
particular action. Generative AI is the converse. It’s creating with these models, whether that be creating images like you can do with a host of services out there. The most well-known one is Mid Journey.
I can go in and prompt it to say, Give me an image that represents Artificial Intelligence regulation or give me an image of a generative AI model creating an application. Oh, and by the way, if you could do this in the style of Picasso. So, the architecture behind these models is similar, but it requires much, much larger models, much more data. They’re much more computationally intensive, and they can still do those things that went before. So, these models are generative AI models, but you can use them for prediction and analysis as well. It’s just that before, everything that we had that we were trying to use for generative use cases was really, really crappy. I mean, it just did not do a good job of it.
It was not plausibly at all human sounding versus now, these models, you go in and put them in front of the Turing Test, which is, can a person tell whether or not this is a model or a human? And increasingly we can’t because they are so good at imitating us, which is, amongst other things, the reason why it’s such a challenge in education. Because it’s really difficult to tell whether one of these things is fake or not because you can tell a model to go in and include the kind of flaws that you would make as a high school student.
And that’s the scary part, right? And we’ll talk more about that later. But first, I’d like to make sure we’re all clear on the difference between predictive versus generative AI. I’ll use an example. If I purchase a book on Amazon. I notice that Amazon will immediately say, “Oh, Alicia if you liked this book, then you might like these other books as well.” Or, “Customers who bought this same book, also bought these books as well.” Is that a form of predictive AI or no?
Exactly. But that structure of that problem applies to just so many decisions that we have in life. So, if I, for example, wanted to comb through all of the emails that have come into my organization and find out, well, which one of these customers is most unhappy and is most likely to leave my company, that is actually a prediction problem. We might not think of it as prediction, but we are predicting the degree to which this email corresponds to that kind of a problem. Or similarly, which one of these customers is most unhappy, or, for that matter, most happy. That’s actually a prediction problem. But it is, as we were talking about before, it’s more of an analytic exercise. It’s trying to understand all of this incoming data that’s coming in.
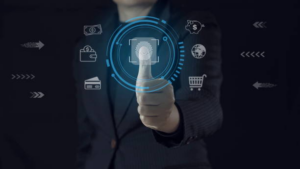
What we have the opportunity to do with generative AI is to flip that on its head and now go in and do it the opposite way, which is, I want to now create that content. I would like to create those emails. I would like to create that code. I would like to create those queries, those visualizations, those presentations, all of those things I would like to create using these models that previously I couldn’t do. I think one of the things that you’re asking is, Well, so what should we use these things for? Should we be going in and looking to replace people with these? Because now it can do these humanlike activities. And for the most part, the answer to that is usually, no.
I have seen very few instances where anybody has lost their job off of this. Is it the case that maybe you need a lot fewer people? Possibly. But for the most part, this is giving human superpowers. It is going in and doing the manual effort, the things that don’t require that much in the way of human intelligence and giving us the head start. So, I often like to think of, like, Clay Christensen had his Jobs to be Done framework and think of what you’re offering as if it was being hired to do a job. What would that job be? For generative AI, I’ve found about five different core jobs.
The first one is the First Draft Generator, creating that first draft of the email, creating that first draft of an investor presentation, creating a first draft of a conceptual graphic, or creating the first draft off of code, or creating that first draft of that graph or chart that you want to display. Generative AI is really good at getting you to that first draft, sometimes even second or third draft. But then the human expert takes over and goes in and tailors it, improves it, does the sense check on it, make sure that it’s factually accurate, because these models do “hallucinate,” which is they will invent facts because that’s what they’re doing. They’re inventing things much in the same way that humans do, by the way. So, a core role is in this First Draft Generator.
A second one would be Information Summarizer, because these technologies are really good at going in and looking across reports, documents, structured and unstructured data, and going in and point and making sense of it, helping me find, So, I’m really interested in this subset of customers because I want to develop this new product. So which of my customers is most likely
using our product in this particular way? And then we can comb across all of the information that’s out there, or which companies that are out there in their financial reports are most interested in this particular topic that my business is going after.
Again, these technologies are really good at combing through all of these documents, reports, online information, and going in and synthesizing not just which reports have that information, which parts of those, but even drawing trends across them. What are the main things that are most important to businesses today is something that you can absolutely feed into one of these generative models with varying degrees of success, and you also want to give it a lot more information in order to make sure that you get something back.
Okay, so that’s two core jobs that we can use generative AI for. But what are the other three?
Other ones are things like Customer Listener going in and listening to your customers as well as actually employees and finding out, so what are they talking about, what are they frustrated with, what do they want the most, et cetera. And then there are two other ones. One of them is like an Application Enhancer. As you’ve already discovered, pretty much every business application under the sun is feeding in generative AI capabilities, usually in the form of some virtual assistant that relies on generative AI to do that. And last but not least, these models are actually really good at Generating Data. Whether that be because you want to protect sensitive data, you want to kind of hide it and obscure it, or whether or not you want to help clean the data in your database by going in and filling in, sanitizing, and structuring your data more correctly, these technologies work pretty well for that.
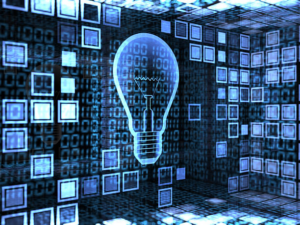
I love the way you just distilled the uses of generative AI into five main roles. So there’s First Draft Generator, Information Summarizer, Customer/Employee Listener, Application Enhancer, and Data Generator. It seems to me, Kjell, that in order for generative AI to work there has to be a source of data that it draws from. For instance, my understanding of Chat GPT is that it scours the World Wide Web, synthesizes data that it finds, and
then provides you a response to your query or command. Is that how it works?
That’s how it works,
Okay.
But there’s a key difference and that’s that whereas previously you pretty much needed to own all of that data to begin with in order to really get started. So, you needed these huge corpuses of data that somebody had gone in and annotated so that the patterns that you were looking for were marked in that data. What’s happened now is that those models are then being made
available to folks that you can take that model and then do fine-tuning on it. So, whereas that large language model that’s out there, there are open-source ones like Falcon is probably the best known, Meta has its Llama 2 that’s out there, and there’s an ecosystem of open-sourced generative AI models that are out there.
You can make them even more accurate by then doing additional fine-tuning on them with your own data or with industry-specific data to make it even more accurate. So, what’s happened is that you can sort of build on the shoulders of giants, as you will and take these models which have already been trained on incredible amounts of data and then go and provide additional fine tuning on them. And sometimes it spans domains in ways that you would have completely not predicted. So right now, most of the pharmaceutical firms that I’m speaking with, for example, are taking these models that are trained and understand human language and then they’re doing fine tuning on molecular data and all of a sudden you now have models that can go in and propose synthetic proteins that you would use for treating things like diabetes and heart disease off of them.
Wow!
It’s not easy by any stretch of the imagination, but there’s something about the commonality in the type of data that we’re looking for and the type of problems that we’re going after that mean that you can take these so-called foundation models and then go and apply them in all of these different domains in ways that we never really thought possible. You can do fine tuning on them. The other thing that you can do is you can feed it a lot of contexts beforehand. I can go in and when the query comes in, say, “Well, this is what the person asked. But I know that they’re in finance. I know that they’re really interested in applying for a mortgage. I want you to give them this information or take into account this information. So, use all of that when you’re then processing this answer and craft a much more tailored answer.”
In these cases, unlike the first one, where you’re taking the model and you’re building on the model here, you’re just using that very generic general-purpose model. It’s just you’re giving it a lot more context and a lot more information to help it customize its output for the specific use case that you want to go for. And that aspect of it is also new. So, you’re building on the shoulders of giants and you’re able to use this multifaceted tool by going in and I don’t know, it’s a little bit like putting the Lego around that tool in order to make it into the specialized tool that you would like.
It’s all good Kjell, I think I understand what you mean. But just to be clear can we use a fairly generic example based on something that relates to everyone who operates a business? We’re all in business to make money and it takes having customers or clients to make that happen. So, let’s talk about, hmm, Salesforce. It’s a CRM designed to keep a list of customer or client data, right?
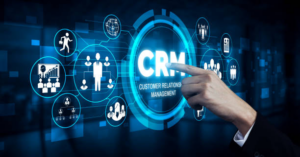
Is it possible to have a generative AI application built that would combine data from Salesforce with, let’s say, online reviews data from sources like Google and TrustPilot as well as email data from a tool like MS Outlook? I’m envisioning an AI-based solution where all of this customer data can be aggregated to give a business owner insight into customer preferences. Those insights could in turn spark new innovations or improved service delivery. Does this example explain, at least in theory, what the possibilities are?
You’re spot on! when it comes to things like Salesforce. You can think of all of that data that’s trapped in those freeform notes that are there, or you can think of all of those emails that are attached to customer interactions, depending on who you’re leveraging. You might also even have transcripts of calls that are in there from outbound sales calls or that are coming in customer service calls. All of that information is there and usually completely ignored because you’re usually forced to read through all of it. The notes were badly written. They’re all inconsistent. And what generative AI enables you to do is to go in and leverage the AI to do that hard work of reading through all of those and extracting out those insights and drawing those trends and finding those needles in the haystack for you.
Salesforce, for example, has Einstein GPT, they have their own large language model that they’re making available. And you can use that to design a still fairly basic generative AI application in Salesforce. But it also tends to be then limited to the Salesforce ecosystem or anything that you can get in Salesforce. And it tends to go after the lowest common denominator, if you will, in terms of what folks will need. Salesforce, much though I love those guys, they’re usually so conservative in terms of actually launching these ones.
That is often a frustration there. And so, what about those other use cases that you’re describing? What about all of those emails that aren’t in Salesforce? What about those reviews that are on Amazon, that are on Walmart.com, Target? What about all those social media posts that are out there? What about those images and things on Instagram? I would love to be able to analyze those. The incredible thing about this technology is that it does open up all of those channels for analysis and makes it scalable and easier like never before. The problem is that it’s not necessarily easy. The applications – people haven’t built enough of that for you. Or if they have built it right now, they’re charging you a lot of money for it, and they might not be leveraging the latest technologies for it. So right now, you’re still a little bit limited in terms of, well, do I have to go hire a data scientist?
Do I have to go in and do the hard work of building one of those applications myself? And often you are, but that’s sort of just a matter of time. The really exciting development going forward is that if you take one of these models. They can analyze and they can generate, but they can’t really take actions today. Say you wanted to go in and create a new item in Salesforce off of a conversation that you that you had.
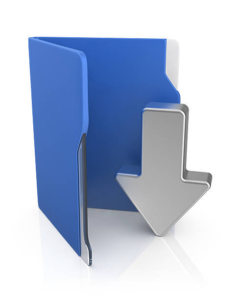
Say you wanted to go in and search a couple of different investor reports that are out there. Download those ten KS from all the industries, from all of the companies that are in this financial services industry that are based out of Massachusetts and go and find for me the ones that are most interested in generative AI and draft an email. Find the key executives that would be most related to AI and draft me a template email for me to go and send and reach out to them. And maybe if you can find their email addresses as well, that would be great. Where people are creating these combinations of generative AI and rules engines that enable you to do that. It still is early days.
But I would imagine there are some off-the-shelf type tools out there right now with some of these capabilities, right?
There are things out there, like Auto GPT that do this, where the model itself will propose a set of actions. I want you to go in and create a business proposal. The model says, “Well, these are the things that are usually in a business proposal. Let me go and I think I should scour your website and go in and figure out what the most important things are in that.” And then next step would be to go in and create that presentation for you. Does that sound like a good idea? And right now, they don’t always work the way that you want to. They especially don’t work the way that you necessarily want to if you let it go and do its own thing without requiring it to go in and get your feedback on it. But that’s the state of the art.
Are you optimistic that the technology will get there?
It’s one of those things where this wasn’t feasible three months ago. For all we know, by the same time next year you might have those kinds of virtual assistants that enable you to do those things. Things that you would normally have required your own assistant to go in, or certainly would require a lot of research and hard work on your part. And now it’s more of a question of
being able to take that problem, figure out what the problem was, break it down, go in and provide that ongoing guidance to one of these models, and then figure out, okay, well, how am I going to improve on what it came back? And we’re going to get to that world extremely quickly.
Which is, I think, great news for small business owners, because small business owners, we don’t have the luxury of people just hanging around who can go in and do that legwork for you. You only have the experts who then have to do everything themselves. And so right now it’s a little bit more limited to, well, I can consume these generative AI models within my existing business
applications so I can go in and leverage those models myself. I can go in and use Bard or Chat GPT or GPT 3.5 or 4, and I can use those to create this content that I want to use, whether that be my email campaign or a proposed marketing campaign or my next business logo or graphic. I can use those myself today. And absolutely everybody should. It is much easier to use them.
I wouldn’t say it’s not foolproof. It’s something that anybody can learn within a couple of hours. So, using those yourself, absolutely. But what’s really exciting is what’s coming down the pike. But you don’t have to wait very long. We figured out something core and now that innovation is happening in weeks where that was taking years or decades before.
Continuous improvement and innovation, right? Kjell, you just said a word that really stood out for me and that’s “foolproof.” This generative AI technology, at least as of today, as good as it is, it isn’t foolproof. Can we, should we, trust the results it provides?
In short, you have to be very careful. You can sort of imagine this when you’re talking to a small child. The perception of what’s real and what’s not real and what things that they’ve made up is always an omnipresent worry. And that is absolutely the case, especially with the generative AI models that we have access to today. They will quote unquote hallucinate, which is they will
make up facts, they will make up people. So, whatever it’s creating, you do need to go and check with a fine-toothed comb to make sure that it’s actually accurate. It comes with the territory.
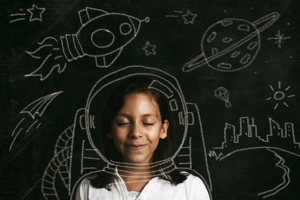
When you’re in this creative realm, it is creating these things for you. It doesn’t distinguish between whether it’s creating a fact or whether it’s creating a description for you. There are ways in which this is being addressed. In the spirit of that innovation, Bard from Google just recently did things like it’ll highlight in its particular things, and it will go and do a separate set of Google searches to see whether these are backed up by Google searches. So, it can speed up that process of fact checking the things that it’s coming up with. So that’s a big thing to be on the lookout for.
The other thing is to be very careful about any sensitive data that you are providing to these models, because especially the free versions that are out there, they are often very explicitly collecting the data that you feed it to use as training for the next versions of those models. So, if you go in and say that you want to write this email to this particular person who is of this particular age, gender, ethnicity, lives in this particular place. Voila! You’ve just sent potentially off something that is PII [Personal Identifying Information] data. And it’s very easy to do, it’s very easy to forget that the terms of use around these models is often very loose for yourself, but also for anybody you’re working with. You want to be informing them of just what you need to be careful about the risks that you run there.
Knowing the risks that are involved, and the fact that this technology can unfortunately be used for nefarious purposes, I’m curious – what do you, Kjell Carlsson, see on the immediate horizon for AI?
Well certainly that connection to action. So that it’s not just something which is analyzing something, but something which is enabling you to take action. So, we hinted very briefly at the fact that some of these services are offering plugins where you can then connect to third party services. Now they’re still very limited. There are things like, well, you can connect to Expedia and so maybe you can use generative AI to go in and find your flight tickets, plan a vacation and do things like that. But that’s kind of at the end of the day, that’s not what we’re really excited about.
The real excitement happens when we start connecting these things into my accounting system. Now I would like to be able to automate more of those things. I can pull out insights from those or having better connectivity into CRM solutions, into ERP solutions and things like that. So, there is this bridging the generation and insights to the action gap that’s happening and
creating these virtual agents or virtual assistants that we talked about before, whereby I can’t hire a whole bunch of college students that I would like to go in and find this information for me and go in and make sense of it. Now I don’t need to.
And what about the proliferation of business applications out there that are using generative AI to in some way enhance their offerings to their customers? You mentioned Pandora’s box being opened earlier, but it’s more like the genie being let of out of its bottle, right?
This explosion of applications with generative AI components of it, we’re going to see it even more. But what we should be talking about is that other aspect to it. But what about the downsides for this? And it is one where, in good news, these technologies are very powerful, bad news, these tools are very powerful. It does make it possible to create fake information to imitate somebody who sounds like a parent, a child. If you haven’t already seen much more sophisticated spam and phishing attacks, you are going to be seeing them soon.
We’re already seeing instances where banks are being so far usually catching them. But AI is being used to generate the voice of a long-term customer. And so, the person on the bank side thinks that they are working with a client who they have authority to go and execute trades for and low and behold, the person on the other side is really a synthesized voice which was just too difficult to distinguish from the real one. All of that’s happening, we shouldn’t forget that obviously next year is an election year and we can expect plenty more deep fakes of fraudulent images, fraudulent statements, you name it, that will almost certainly be coming.
There are, without doubt, worries and challenges on that front that we’re going to much in the same way that I think our parents’ generation didn’t really need to worry about phishing attacks in the same way, or the Nigerian print scams and things like that. We’re going to have another generation of those far more sophisticated and we’re going to be teaching our kids as to what are the ways in which you can spot those.
These are definitely things we should all pay attention to as we adopt this technology and use it to streamline our operations. As we start to wrap up here Kjell, can you let everyone know the best way to contact you? And do you have any parting words of advice when it comes to AI adoption specifically for business operations?
Well, please absolutely reach out to me on LinkedIn. There are very few Kjell Carlsson in the AI space out there generally, so don’t hesitate to reach out to me directly. I’m also the host of the Data Science Leaders podcast. If you feel inspired to find out about the lives of those miserable folks who are on the hook for going in and delivering outcomes with these things, please don’t hesitate to tune into that. We also do have a host of resources on our Domino Data Lab or Domino.AI website. For example, I have a report coming out on low hanging Generative AI projects and talks a little bit more about those five jobs to be done with Generative AI.
But there is so much good content that’s out there. I am really excited to be hearing the rest of your season where I think you are going to be demystifying these things even more and are going to be supplying even more practical guidance to folks. More than we can go into in this short time. But above all, I would say do not fear getting started with these tools. Sometimes it can be a little bit intimidating, sometimes it can be easy to get on them. There is so much that you can do today with these tools to increase your productivity. You would really be missing out if you’re not taking advantage of them.
Heed Kjell’s words everybody! And on that note, this concludes our fireside chat. What a great way to kickoff our AI Audio Conference! Thank you, Kjell and I hope you stick around for the rest of the conference!
My pleasure. I’d be more than happy to.
Please, please!
Thank you very much, Alicia!
Thank you for listening! If you enjoyed this episode, then please subscribe and give us a five-star rating and review. You can find links to the resources Kjell mentioned as well as the video version of this fireside chat at BusinessInfrastructure.TV. That’s BusinessInfrastructure.TV.
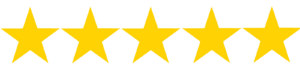
We hope you enjoyed this primer on AI. As a recap, we covered what AI is as a subset of machine learning, why it’s really all the rage now, and how it can revolutionize the way you operate your back office and cure those operational blues.
Down the hall is our next speaker. He’s going to give us our first AI product demo during this conference.
Be sure to come back to the place wherever you’re listening to this episode so you don’t miss it! Until then, remember to stay focused and be encouraged. This entrepreneurial journey is a marathon and not a sprint.
This podcast episode was written and produced by yours truly, Alicia Butler Pierre. Kenya A. Moses provided the voiceover for the conference’s intro. Audio editing by Olanrewaju Adeyemo. Original score and sound design by Sabor! Music Enterprises. Video editing by Gladiola Films. A special thank you to our sponsor, Equilibria, Inc., to Erika Ve Revilla for creating the show notes, and to Monique Inge, our guest relationship manager.
This is the Business Infrastructure – Curing Back-Office Blues podcast.